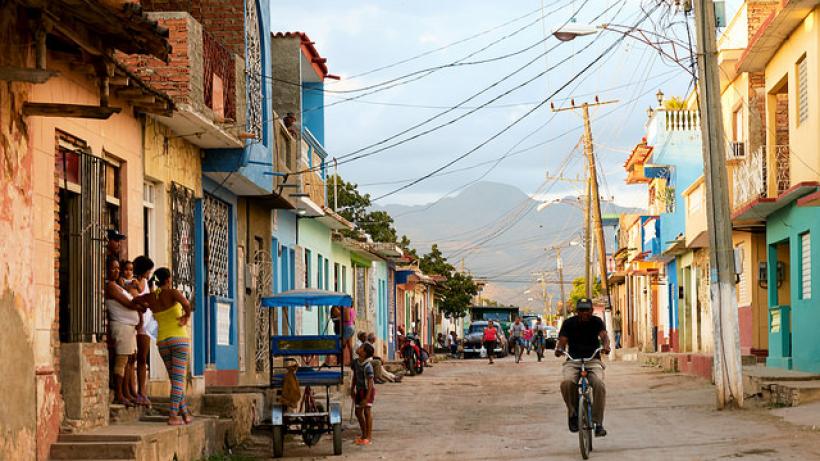
Fighting poverty with big data: A conversation with Joshua Blumenstock
Joshua Blumenstock is an Assistant Professor at the UC Berkeley School of Information, where he directs the Data-Intensive Development Lab, and a member of the Blum Center’s Development Engineering faculty. His research lies at the intersection of machine learning and development economics, and focuses on using novel data and methods to better understand the causes and consequences of global poverty. Blumenstock has a Ph.D. in Information Science and a M.A. in Economics from U.C. Berkeley, and Bachelor’s degrees in Computer Science and Physics from Wesleyan University.
What can remote sensing and geographic information system data and cell phone data tell us about a person living in poverty?
Blumenstock: We have partial answers to that question. The work that has been done indicates we can estimate very basic things: population density, household average wealth, basic indices of relative socio-economic status. Of course, there are lots of different ways to measure poverty and inequality and welfare. People working in developing countries tend to like consumption because it seems to be most closely correlated to how someone is actually doing. There has been some work looking at whether you can estimate consumption and expenditures from remote data sources, and initial results are promising here too. Aside from measuring basic welfare, all sorts of work is being done to use these data to learn about migration, social network structure, and the spread of disease, to give a few examples.
What can data tell us about poverty indicators such as the incidence or depth of poverty?
Blumenstock: What these models actually spit out are sub-regional estimates of welfare. We can define welfare however we want. In general, as long as you can measure it in the traditional way, you can use these non-traditional data and models to try to estimate it. However, depending on what you want to measure, and what data source you are using – such as phone data or satellite data – your estimates may be more or less accurate. But once you have your estimate of the distribution of wealth, you can do all of the things you could do with traditional data. You can back-out the poverty incidence, Gini curves and other constructs you derive from the poverty distribution.
In what ways are these data sources limited in their ability to measure welfare or other things?
Blumenstock: I think we have to be careful because it is easy for people to get excited at the potential applications before really understanding the fundamental and practical limitations. Nonetheless, here are several margins where this could be a major improvement over the status quo. One is cost: it is a lot cheaper to collect cell phone data, for instance, than a nationally representative household survey, which costs tens of millions of dollars. Another is geographic resolution: budgets constrain the areas and number of people that you can survey, but satellites can quickly collect millions of images from a small region. Another is temporal resolution: again, because of the costs, you can only do a nationally representative survey every few years at best, but phone data gets updated every second and satellite data gets updated every day. So, if you can update your estimates of the things that you care about – the poverty incidence or the distribution of wealth – every day, that could be really useful. We can think about all of the applications: not just program targeting and impact evaluations but also programme monitoring and disaster response. All of these things need up-to-date estimates of the distribution of welfare. Those are all of the reasons why I think people can be excited, including me, but we are just at the very first baby steps of that long pipeline.
There are basically two canonical papers out there: one that I worked on and one that a Stanford group worked on. Using the most up-to-date data, what we show is that cell phone meta data can be used to estimate relative wealth very accurately. The group at Stanford shows that daytime satellite imagery can be used to do the same thing. They also look at consumption, and find similar results. However, both of those studies include a very small number of countries at one point in time. We have no idea yet if those models that you calibrate at one point in time can generalise into future points in time. To do a lot of the tantalising applications, like monitoring and impact evaluations, the first step, which has not been done yet, is to show that these estimates can reliably allow for inference over time.
Poverty is heterogeneous across both space and time. Some of your work highlights the ability of machine learning to provide granular spatial assessments of poverty and assessments of poverty in real time. What are the implications of this for the delivery of poverty alleviation programmes?
Blumenstock: I do not think this is going to solve any of these age-old problems relating to the shallowness of quantitative estimates. There is always going to be trade-offs between quantitative and qualitative research. There is the famous quote that says, “Not everything that counts can be counted, and not everything that can be counted counts.” Here, it is no different. There is a latent characteristic of people, which is welfare or well-being, and that is what we want to measure. However, you cannot measure it directly. You can either embed yourself in a community and really get a sense for it, or, if you want to measure welfare at scale, you have to rely on these instruments that make observations about one dimension of things that we think are correlated with this fundamentally unobservable state. And what we are doing is even one-step removed from that. We have these instruments that are already imperfect measures of that underlying state, and we want to try to replicate that using data that we get for free at true scale. At best, what we are doing is trying to replicate those already imperfect instruments, which adds another layer of imperfection.
There certainly is nothing about what we are doing that imposes homogeneity, though. We would never use a model that we fit on Rwanda and apply it to Kenya without knowing first how much we expect the model’s prediction to degrade by. Similarly, I would never expect a model that was fit in 2016 to be accurate in 2018. But that is an empirical question, and one that we are actively studying. Some of these are solvable problems, but I think there are these other unsolvable, philosophical questions like construct validity and if we are measuring that thing that we care about. Those things are more fundamental.
Household income changes overtime due to income shocks throughout the course of a year. How do you view this as a mechanism to correct problems in real time? Could this inform programmes to better smooth consumption and target across time as opposed to just one moment?
Blumenstock: I think there are some very compelling applications that look at inter-temporal consumption or changes in dynamics. For a lot of reasons, you might think that that is a first order thing to target rather than a cross-sectional, stable measure of permanent income. In principle, this is exciting because with this line of research you can potentially get updated estimates at very high frequencies. Yet, that is a couple steps ahead of where we are now. In my mind, step one is seeing if data from a single point in time is accurate. We can sort of do that now. Step two is seeing if the estimates can remain accurate across different points in time. We have not quite gotten there, but we are working on it. Step three is what you are talking about: real-time estimates. I think those need to be done in that order. Unless we know the right way to generate dynamic estimates and, then, know the right way to layer those onto a real time streaming data set, real time estimates will be wrong. That will not be for another few years.
You mentioned that you would not fit a model from one country to another country. What are the ways forward to potentially be able to generalise from one country to another using this data?
Blumenstock: Generalisability can mean a lot of different things. One is generalising over space, like from one country to another or even within one country from one region to another. Another is generalising over time. A third is generalising from a population that you observe to a population that you do not, even if it is in the same space at the same time.
Of the three, I think that generalising from one country to another is the easiest. That is an empirical question. We can collect data from two countries, or 10 countries (I am working on a project where we are collecting data from 50 countries), and we can just see if we train the model in one country and apply it to another. Then, we need to determine if the degradation of the model’s estimates depend on things that we observe: whether the countries are on the same continent or how far apart they are, whether the ethnic composition of the two countries is similar, or if the distribution of wealth is similar or not. These are things that we can measure. So, we do not want to just apply the estimates from one country to another, but rather, we want to have a sense for how to correct for translation errors, or at least know when and where such errors are likely to exist. We will hopefully have answers to some of these questions in the next few months.
We are also actively working on this project to have a sense for the ability for phone- and satellite-based estimates to generalise over time. But realistically, it will be a while until we have a conclusive answer.
The hardest one is generalising from an observed to an unobserved population. That said, there are a lot of techniques from traditional econometrics that you might apply to this problem. If you know the process that governs whether someone is observable or not, then you can “reverse engineer” a statistical correction. For instance, if you only observe people from population A, you can only reliably estimate the distribution of wealth in population A. But, say you want to be able to estimate the wealth of population B, but they are not visible in your data (because they do not have phones, for instance). In this scenario, if you know something about how the distribution of wealth of population A relates to that of population B, you apply a transformation to the estimates of population A to get estimates of population B. These are not big data things or new data things. These are old problems with sample selection and construction, to which we have partial solutions.
What are some of the ethical concerns with using machine learning to track poverty that you are confronting and how do you confront these issues, especially in consideration of the inherent vulnerability of those who your work is meant to serve?
Blumenstock: The thorniest ethical questions for me are more philosophical ethical questions: things like the legibility of populations and the possibility of misappropriation. Is it a good thing to make people easier to measure? What if an authoritarian regime takes the papers that I am writing and uses them to weed out political dissenters? For these sort of questions, I try to look at the scale of negative use cases and positive use cases, and focus on problems where the positives outweigh the negatives.
Another important issue is privacy, in the sense that we often deal with data that people generate without a full understanding of how it can be used to draw inferences about them. Here again there is an ethical concern and a practical one. The practical one is easier to address: at least as far as our research is concerned, we do our work in a controlled research environment. We put in place rigid data protection procedures, like removing personally identifying information prior to conducting analysis, to ensure that, to the extent that we can, we are safeguarding the privacy of the people we study. But we cannot control the privacy practices of others, like industry or government. So, the ethical issues are bigger, and boil down to a similar calculus as I mentioned earlier – do we think the benefits outweigh the risks?
There is an assertion that there is tension between technocratic solutions and human-rights based solutions. Beyond the implications for poverty alleviation, in what ways does or could machine learning contribute to good governance or protection of human rights?
Blumenstock: It could contribute in a lot of ways — both through the applications that have been developed in the last few years, as well as those that are on the horizon.
I can see why these “big data measurement” methods may seem more natural a fit for technocratic approaches. But bottom-up governance structures need data too. You need to know who your constituents are. A lot of the things that we are measuring now are largely motivated by more of the holistic, softer things that have been off limits to technocratic fixes. For instance, we are working on a project now that tries to quantify the extent to which violence disrupts the social fabric of places like Afghanistan — using phone data to observe the social fabric in ways that would not be possible with other methods. Technocrats are limited by what they can observe, and one of the things they have a hard time observing is community cohesion and fragmentation. If we can provide ways for people to more directly observe that then it can create a bridge between what technocrats are equipped to do and the more bottom up approaches to good governance.
This article has originally been published here.